On-Chain Analytics For Predictive Modelling
Oct 28, 2024
5 min read
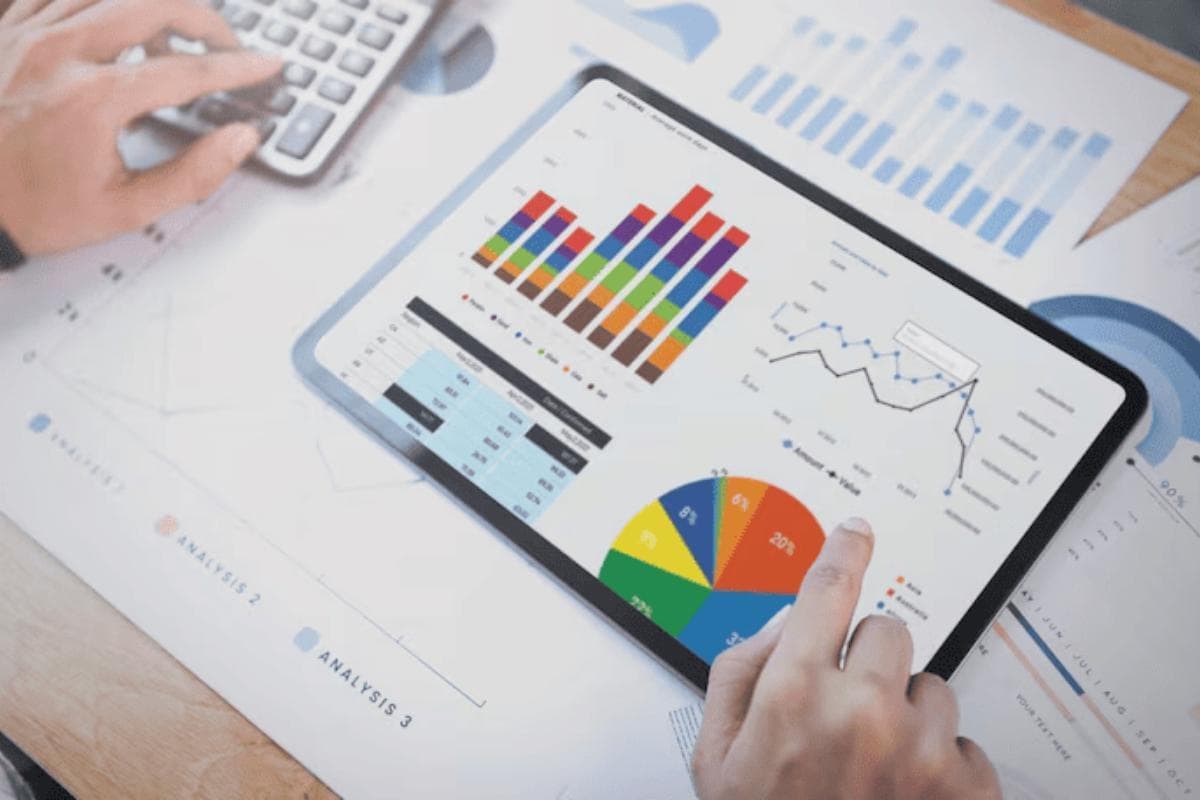
Key takeaways
- On-chain analytics corresponds to a comprehensive study of a blockchain network through past data patterns and trends with various statistical and algorithmic tools.
- There are multiple indicators of on-chain data that are essential for on-chain analysis.
- These include active users, total value locked (TVL), transaction volume, trading volume, token holders, and others.
- The reliability, transparency, and safety of on-chain data make it applicable for use as an input for on-chain analysis.
- As an emerging sector, the blockchain space could do with several use cases of predictive modelling in the future.
Blockchains are decentralised and immutable digital ledgers where all transactions are entirely transparent. This factor has led to potential use cases of blockchains in various industries worldwide. But simply putting things on-chain doesn’t solve business problems - analysing and comprehending the data recorded in a blockchain is crucial for identifying the trends and performance of a particular blockchain and making future decisions backed by data. This process, known as on-chain analytics, can also be critical for predictive modelling in the crypto market.
Important drivers behind the traditional predictive models that one sees today include statistical strategies and emerging technologies. Data, which is the most pivotal input for predictive modelling, needs to be analysed in minute detail to create accurate models. Other tools and techniques like machine learning algorithms, data visualisation tools, or statistical software can also help with on-chain analytics.
Understanding on-chain analytics
On-chain data of blockchains is analysed by popular blockchain data analytics platforms like Token Terminal and Glassnode. ICR’s crypto scoring and ranking product is also a useful tool for studying various DeFi tokens, smart contract platforms, digital currencies, and utility tokens using major on-chain data indicators.
On-chain data indicators are important for on-chain analytics, but are also crucial for making a fundamental analysis of a blockchain.
While on-chain analytics focuses extensively on data patterns and indicators to make data-driven predictive models, on-chain analysis emphasises the fundamental performance of a blockchain through recorded data indicators.
The importance of performing on-chain analysis
On-chain analysis provides a comprehensive picture of a blockchain, both fundamentally and technically. On-chain indicators like market cap, circulating supply, transaction volume, and others are essential for working out the potential and current functioning of a blockchain. An on-chain analysis also leverages the data that is recorded on the blockchain, which is its distinguishing feature because the data is completely transparent and immutable.
On-chain analysis is done to derive a fundamental or intrinsic value of a crypto. It is similar to how investors study a company's quarterly report and balance sheet before studying its stock price. Just like 'daily active users' is one of the key metrics to measure a company, say Instagram's success, similarly, depending on the utility of the crypto, it must be possible to measure its success by identifying certain key metrics.
Thus, on-chain analysis is highly impactful in guessing market sentiments and investment decisions.
Here are some of the most essential metrics for performing an on-chain analysis:
- Active users: Active users refer to the addresses on the blockchain that are regularly engaging and active, either on a daily, weekly, or monthly basis. The number of active users in a blockchain hints at the adoption and engagement in the network. A rise in active users could mean that the blockchain network is experiencing wider adoption. It is measured by calculating the number of unique addresses that have either sent or received crypto.
- Transaction volume: This on-chain data metric measures the total volume of transactions occurring in a blockchain network (send or receive or burn or mint transactions) in a definite time period. Volatility, network activity, demand, and other indicators could be determined with this metric.
- Total value locked (TVL): The total value of assets locked in a blockchain network’s decentralised applications (dApps) and smart contracts is called its TVL. Locking is done by staking, lending, or locking in liquidity pools, etc. The levels of adoption and dApp activity in the blockchain can be estimated with the TVL metric.
- Token holders: All addresses in the blockchain that hold its native tokens, with balances greater than zero, qualify as token holders.
- Trading volume: Trading volume is an important on-chain metric for decentralised exchanges (DEXs), and it underlines the extent of liquidity and commissions or revenue earned by the DEX.
- Burn rate: This term is defined as the rate at which a blockchain protocol reduces the number of tokens in active circulation. This can lead to an increase in its price due to short supply.
- Percentage of assets staked: As the name suggests, this metric is a computation of the share of total assets staked in the blockchain protocol.
- Protocol fees: Blockchain protocols also charge a fee to their users for distributing among miners or validators to incentivise them to run the network and participate in its vision of decentralisation.
- Protocol revenue: The total net revenue earned by a blockchain protocol from all its sources of income is referred to as protocol revenue.
- P/F ratio: Price to fees (P/F) ratio is a valuation metric for tokens, which is evaluated by the ratio between its total market capitalisation (diluted or circulating) and the annualised fees of the blockchain network. This ratio is similar to the P/E ratio of a stock in traditional finance.
Predictive modelling with on-chain analytics
Predictive modelling is already prevalent in traditional financial markets to improve efficiencies and minimise risks. In other avenues, such as weather forecasting, predictive modelling is also done widely based on historical data and trends. Hence, it is proven that trusted, safe, and large amounts of data are needed to create the best predictive models.
Blockchain networks fill all three criteria for data. With immutability and decentralisation as underlying factors, a blockchain can deliver the most committed data that cannot be tampered with.
At the same time, with thousands of nodes (or devices) that validate transactions in blockchains, the sampling sizes of on-chain indicators can be quite large. The presence of cryptographic signatures in blockchain protocols also makes on-chain data extremely safe and reliable!
The future of predictive modelling with on-chain analytics
As we've explained in this blog, on-chain analytics is certainly a catalyst for predictive modelling in the blockchain sector. Such models have many viable applications in crypto, including predicting the price movements of crypto tokens. Predictive modelling can also be used to discover new innovative domains that blockchain projects can explore. All things considered, on-chain analytics and predictive modelling can disrupt crypto!
Dive into the various facets of crypto and blockchain, only with India Crypto Research!
Disclaimer:
The information provided in this blog is based on publicly available information and is intended solely for personal information, awareness, and educational purposes and should not be considered as financial advice or a recommendation for investment decisions. We have attempted to provide accurate and factual information, but we cannot guarantee that the data is timely, accurate, or complete. 1 Finance Private Limited or any of its representatives will not be liable or responsible for any losses or damages incurred by the Readers as a result of this blog. Readers of this blog should rely on their own investigations and take their own professional advice.